Infra-Low Frequency Neuro Feedback Modulates Infra-Slow Oscillations of Brain Potentials: A Controlled Study
Received Date: May 19, 2020 Accepted Date: June 07, 2020 Published Date: June 10, 2020
doi: 10.17303/jber.2020.4.104
Citation:Vera A Grin-Yatsenko (2020) Infra-Low Frequency Neuro Feedback Modulates Infra-Slow Oscillations of Brain Potentials: A Controlled Study. J Biomed Eng 4: 1-11.
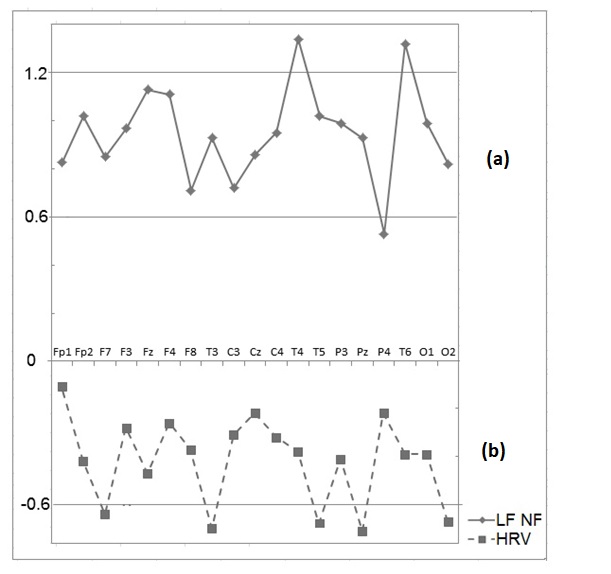
Abstract
A formal comparison of Infra-Low Frequency Neurofeedback with an active control condition, Heart Rate Variability training, is undertaken in the present research. 17 participants 21-50 years of age with no history of neurological or psychiatric diseases conditions, but reporting about some physiological or psychological complaints were involved in the study. Participant progress was monitored by means of Visual Go/NoGo test performance and spectral power of slow EEG oscillations during the test before and after twenty sessions of training. Outcomes favored Infra-Low Frequency Neurofeedback training over Heart Rate Variability training with respect to health status and Visual Go/NoGo test results. Significant elevation in amplitudes in the Infra-Low Frequency range was observed only for the Neurofeedback cohort.
Keywords:Neurofeedback; Electroencephalogram; Infra-Slow EEG Oscillations; Heart Rate Variability; Infra-Low Frequency Training
Introduction
Infra-slow rhythmic potential oscillations were first studied extensively in animals by the Russian neuroscientist Nina A. Aladjalova [1]. This work first became accessible to English language audiences through her book titled “Slow Electrical Processes in the Brain” [2]. Western neuroscience had gone dark on the tonic Slow Cortical Potential (SCP), dating from the time in the late thirties when tube amplifiers required AC-coupling for extraction of the EEG signal. When interest was revived in the sixties, it was with an exclusive emphasis on the phasic SCP (Contingent Negative Variation, Bereitschaftspotenzial). Interest in the tonic SCP only revived once researchers took an interest in the slow fluctuations revealed in the blood-oxygen-leveldependent (BOLD) signals of functional magnetic resonance (fMRI) [3,4]. The hemodynamic response of the BOLD signal limits the accessible bandwidth to < 0.1 Hz. Recently published full-band EEG (fbEEG) recordings raise awareness of the value of previously neglected infra-slow oscillations (below 0.5 Hz) in neuroscience research [5].
Despite decades of residing in scientific backwaters, infra-low frequency (ILF) phenomenology was extensively studied by some distinguished scientists [6-10]. The substantial role of ILF in the spatiotemporal dynamic organization of the brain had been documented long before the first publications on the significance of the ILF surface potential in revealing the internal regulation of the Default Mode Network [11].
ILF potentials identified in different electrophysiological settings are obtained by surface electrodes or by electrodes implanted into various cortical and subcortical structures. ILF revealed in in vivo studies shows correlated behavior when recorded simultaneously viafbEEG [12,13] and fMRI [14,15].
The close relation between ILF, BOLD, and the Monochromatic Ultra Slow Oscillation (MUSO) identified in EEG recordings in the vicinity of 0.1 Hz suggests a relation between these signals and cerebral vasomotor fluctuations [16]. This assumption was supported when researchers were able to detect causal coupling between low-frequency EEG oscillations and well-known Mayer's waves observed in the blood pressure, heart rate variability, and blood oxygenation level distributed around 0.05-0.15 Hz with a peak at 0.1 Hz frequency range [17-19].
Extensive research revealed a significant role of the ILF regime in regulating cortical excitability [20]. The ILF regime dominates the dynamics of large-scale brain organization [21-23]; and it reflects interactions between distinct functional networks throughout the performance of cognitive tasks [11].
Deviations from expected ILF potential distributions have been identified for ADHD, Schizophrenia, Autism, Depression, Anxiety, and other brain disorders [24-29]. These data support the hypothesis of impaired ability to preserve an optimal level of cortical activation in psychiatric disorders [30, 31] and are in line with the assumption of diminished flexibility and the capacity to recruit functional networks during action preparation and performance [32].
Brain activity can be modulated in real-time by way of neurofeedback, a relatively passive brain-computer interface approach that allows the trainee to alter brain function through feedback on brain potentials derived from surface electrodes and presented in the form of visual, auditory or tactile signal streams. In ILF neurofeedback the primary driver is feedback on the surface potential below 0.1Hz [33, 34]. It is called ILF neurofeedback (or ILF training) because the process was found to be highly frequency-specific.
ILF training was developed by Susan and Siegfried Othmer in 2006, as described in a paper titled Clinical Neurofeedback: Training Brain Behavior [35]. The method has demonstrated positive outcomes for a variety of mental conditions, including Post-Traumatic Stress Disorder among military veterans [36], different forms of anxiety, depression [37], sleep disturbances, ADHD, the Autism spectrum, developmental trauma, migraines, and other headaches, epilepsy [33] and traumatic brain injury [38,39].
ILF training can yield surprisingly substantial improvements in a variety of clinical conditions in a reasonable number of sessions (20-40). The tonic slow cortical potential appears to be a direct reflection of the dynamics of cortical excitability. With a signal derived from bipolar montage, network relations are revealed, and thus the training impinges directly on dynamic functional connectivity, in addition to calming hyper-excitability directly. By operating in the ILF regime, the training preferentially accesses the functional connectivity of the intrinsic connectivity networks that were originally identified in fMRI [40].
ILF training also impinges on the ultradian cyclic fluctuation of physiological arousal, as well as autonomic nervous system regulation [10, 41]. The data of Smith and colleagues, employing an adaptation of the method used here, supports the hypothesis that ILF training preferentially influences autonomic nervous system regulation and thus improves the emotional equilibrium of patients, which in turn positively influences attention and working memory [39]. Further evidence with respect to vigilance and attention was recently documented in a large-scale compilation of pre- and post-training continuous performance test data on a clinical population [42]. Clinically significant improvement in performance was consistently observed among those in deficit, largely irrespective of the clinical condition being targeted in the training.
Heart rate variability biofeedback (HRV) is another method widely used to improve stress tolerance and quality of sleep, as well as to reduce anxiety, depression, and other symptoms. HRV is a complex signal that involves a mixture of superimposed oscillations, triggered by reflexes and modulated by autonomic pathways [43-47]. The HRV signal can be segregated into two main frequency bands. The low-frequency domain (0.01-0.15 Hz) is governed by sympathetic activation (vasomotor and baroreceptor origin) and the high-frequency domain (0.15-0.45 Hz) reflects parasympathetic or vagal influence (corresponding to respiration) [48].
The autonomic nervous system (ANS) balance reflects the organismic ability to adapt to internal and external stressors, and HRV is a good biomarker to assess autonomic balance. Elevated stress causes an increase in heart rate and decreased HRV (augmented sympathetic load, whereas increased HRV is associated with decreased heart rate and augmented parasympathetic load (decreased stress reaction).
Deviations in HRV patterns have been reported for patients with anxiety disorder, depression, and insomnia [49-51]. For these disorders, deficiency in autonomic regulation has also been reported [52]. HRV biofeedback is based on the observation that parasympathetic activation can be modulated by simple breathing techniques, with breathing frequency optimally in the vicinity of 0.1 Hz. Improved autonomic balance is reflected in higher amplitude heart rate oscillations centered on the breathing frequency
The present study design included both ILF neurofeedback training (ILF NF) and HRV biofeedback training for purposes of comparison. Both techniques generally improve autonomic regulation. However, since biofeedback is cognitively mediated, it is subject to greater ambiguity with respect tospecific effects on ANS balance. Moreover, it impinges on brain functional organization only indirectly [48].
The goal of the present study is to compare the effects of these two biofeedback approaches on ILF spectral components in order to demonstrate that the ILF training procedure induces persistent changes in the amplitude distribution within the ILF spectral range and to document improved outcomes with respect to the control group undergoing HRV biofeedback training
We postulated that HRV biofeedback at Mayer's wave frequency would be a reliable control in order to clarify whether the spectral parameters in the ILF regime are substantially altered during ILF NF, or, alternatively, the effects of training are predominantly seen in the autonomic nervous system. In the latter case, HRV and ILF NF would be expected to yield similar outcomes.
Materials and Methods
Participants
Seventeen healthy individuals voluntarily participated in this study. With randomization, nine were assigned to the ILF NF (main) group (6 males and 3 females, ages 21-50 years, mean 33.1 years), and eight were assigned to the HRV BF (control) group (3 males and 5 females, ages 23-49 years, mean 35.9 years). Subjects were recruited through the St. Petersburg State University’s and Pavlov First St. Petersburg State Medical University’s participant pool, scientific staff of the Institute of the Human Brain of the Russian Academy of Sciences, and by word-ofmouth.
In order to qualify for the study, participants were required to complete self-evaluation forms documenting physiological, functional, and psychological parameters of health, and anamnesis of vitae. Participants were excluded if they had major chronic health problems; complicated perinatal period; abnormal mental and/or physical development; head injury with cerebral symptoms; the history of convulsions; neurologic or psychiatric diseases; current medication or drugs. Despite the fact that none of the participants had any medical diagnosis, some of them reported mental or physical complaints, e.g. symptoms of anxiety, episodes of depressive mood, mood swings, high reactivity to stress factors, fatigue, headaches, and sleep problems. Several participants were not satisfied with their memory function and/or concentration.
No specialized instruments were used for the assessment of physiological and psychological parameters in this study. First of all, the complaints were heterogeneous and minimal in most participants, and secondly, the clinical effects of the ILF NF were subsidiary to the influence of ILF NF on the infra-slow frequency potentials for purposes of this study. The research was conducted in accordance with the Declaration of Helsinki. All participants gave informed consent after a detailed explanation of the procedure.
The baseline investigation was performed 1-7 days before starting the course of training sessions and consisted of quantitative electroencephalogram (qEEG) recording while under a mild cognitive challenge of a continuous performance test. qEEG parameters were analyzed by comparison against the Human Brain Institute (HBI) normative database. There were no significant differences between groups in any variable. The test was repeated after 20 sessions in 1-7 days after the last session. The results of the second test were statistically compared with the pre-treatment baseline.
EEG investigation
The electroencephalogram was recorded with the Mitsar 21-channel EEG system (Mitsar, Ltd). 19 silver-chloride electrodes were applied using Ten20 conductive electrode paste according to the International 10-20 system. The input signals referenced to linked ears were digitized at a rate of 250Hz, band-pass filtered at 0.01–50 Hz, and notch filtered at 50 Hz. The ground electrode was located on the forehead. All electrode impedances were maintained below 5kOhm. EEG was recorded during the performance of the visual cued GO/NOGO task [53]. Quantitative data were obtained using WinEEG software.
Epochs with an extreme amplitude of un-filtered EEG and/or excessive slow and high-frequency activity were automatically excluded from further analysis. Eyeblink artifacts were corrected by setting to zero the activation curves corresponding to eye blinks. The method is consistent with the one described in Vigario [54] and in Jung et al. [55] Continuous artifact-free EEG epochs were selected for analysis manually. Epochs duration varied among the subjects from 550 to 1100 seconds. The Thomson’s multi-taper method was used to determine the average spectral density in the 0.01 - 0.5 Hz frequency band for each electrode site, for each subject and for each condition separately. Data were logarithmically transformed prior to statistical analysis.
Neurofeedback
For ILF NF we used the Cygnet system (BEE Medic), which consisted of the NeuroAmp II amplifier and Cygnet software, integrated with Somatic Vision video feedback installed on a standard personal computer (PC) running a Windows 7 operating system with a high-resolution monitor. For each of the nine NF group subjects, the optimal reinforcement frequency (ORF) was determined at the beginning of each neurofeedback session, based on the trainee's subjective report. Participants in the NF group were instructed to observe their physiological state, their level of vigilance and alertness, and their emotional ambient while watching a videogame on the monitor and to report to the therapist about felt changes in their state. The training was started with bipolar placement at one or both of two initial placements, T4-T3 and P4-T4 (according to the International 10-20 system), to observe the trainee's response and, on that basis, to guide further optimization. Later, T4-Fp2 and T3-Fp1 electrode locations were added to the protocol as needed. This choice of electrode placement and their sequencing has been standard in the Othmer Method for ILF NF for some years [56]. Each participant received 20 separate 30- to 45-minute neurofeedback sessions over a period of 7–8 weeks.
HRV biofeedback
HRV BF for 8 participants of the control group was provided by the emWave PC Stress Relief System designed by the HeartMath Institute. The plethysmograph placed on the participant’s earlobe furnishes the information on his/her heart rhythms to a software program that provided real-time feedback on their performance. They were instructed to use deep, abdominal, or diaphragmatic breathing, approximately 5-7 complete breath cycles over the course of 1 min, following the techniques learned with the emWave program. The graphics displayed on the computer monitor depicted the HRV power spectrum. Three bars of varying colors reflected coherence, corresponding to low, medium, or high coherence levels. Participants practiced achieving a higher level of coherence relative to the basic level, an objective usually met without difficulty.
Sessions were 30 min in length, and they occurred 2-3 times per week. Each subject received 20 separate HRV BF sessions over a period of 7–8 weeks.
Statistical analysis
One-way ANOVA with factor condition (before – after) for all locations (19 electrode positions) was used to ascertain the statistical significance of the training effect on the slow oscillations for each group. The same ANOVA test was used for the difference of logarithmical power of slow EEG oscillations with the factor (main group-controls) to assess the statistical significance of changes after the training course between the two groups. To quantify the effect size of differences of EEG spectral power Cohen’s d was computed for each electrode position separately. A Cohen’s d of 0.6 was adopted as the threshold of significance.
Results
After completion of 20 training sessions, all nine participants of the ILF NF group indicated improvement in their health status. Most of them reported a decrease of reactivity to stressful factors and release of inner tension, improved body, and spatial awareness, and mood stability. Also, they noticed an increase in energy level and better cognitive performance.
Six of eight participants of the HRV NF group also reported positive changes in their health status after finishing 20 sessions. The observed improvement consisted of increased stress tolerance and a better ability to relax. Two participants of the control group were not sure that the training course induced any notable changes in their state.
The results of Visual Go/NoGo test performance were as follows: Of the 9 participants in the ILF NF group, 2 made no errors pre or post. Of the remaining seven, 4 showed improvements in both omission and commission errors, while the remaining three declined in performance in one or another of the categories. In the control group 4 of 8 participants made fewer omission errors, and 2 of them – fewer commission errors. In 3 subjects an increase in the number of omission or commission errors was observed, and in 2 of them, the results did not change. Whereas the individual data are too granular to draw conclusions, the cumulative data do indicate a trend favorable to neurofeedback. Total commission errors declined from 14 to 1 in the NF group, versus a decline from 5 to 4 in the BF group. Omission errors declined from 60 to 20 in the NF group, versus an increase from 15 to 24 in the BF group.
The mean Response Time (RT) declined by an insignificant 2% in both groups, whereas the mean Response Time Variability (RTV) improved by 7% in the NF training group and by 13% in the BF group. The difference was not significant. In the final analysis, no significant group differences could be identified in the Visual Go/NoGo test data.
In 13 out of 17 participants (77%), the baseline EEG contained rhythmic infra-slow oscillations in the frequency range of 0.06–0.12 Hz against the background of the prevailing slower potential fluctuations. The localization of these oscillations varied: in some subjects, the episodes of rhythmic 0.06-0.12Hz activity were widespread, without any definite local dominance; in some cases, these fluctuations were observed over the frontal-central region, while in other cases they were found over the posterior brain areas.
The post-training EEG patterns in all 9 members of the ILF NF group revealed a significant enhancement of spectral power in the 0.01-0.5Hz frequency band compared to the pre-training EEG. One-way ANOVA detected a significant main effect of the factor "Condition" for the slow activity in 0.01-0.5Hz frequency band for the ILF NF group: F [1,16] = 5.22, p < 0.04. An increase in average power in the 0.01-0.5Hz band is seen in all 19 electrodes locations. For the control group, the average values of the power of slow oscillations in the frequency range 0.01–0.5 Hz decreased in all locations after the training, but this decrease was not statistically significant F [1, 14] = 0.96, p <0.35. These effects are illustrated in Figure. 1a, b.
The effect size for changes in the logarithmic power in the 0.01-0.5Hz band was therefore higher for the main group compared to the control group for all electrodes. These effects are illustrated in Figure. 2. In the control group, changes in slowwave activity after the course of HRV BF were not statistically significant
One-way ANOVA test with a factor (main group-controls) revealed the statistically significant difference in the change of power in the 0.01–0.5 Hz frequency band for the main group compared to the change for the control group F[1, 15]=10.65, p = 0.005.
Along with the general enhancement of spectral power in the 0.01-0.5Hz frequency band in the main group, rhythmic fluctuations in the 0.06-0.12Hz band became more prominent in 8 of 9 subjects. It should be noted that the localization and peak frequency of oscillations changed in some cases with the training, but no general trend was observable. In the control group, the power in the 0.06-0.12 Hz band increased in 3 of 8 participants and did not change or even decreased in some degree in 5 of them
Discussion
Infra-slow oscillations with frequencies below 0.5 Hz are observed in the human brain in form of 1) fluctuations of electrical potential measured with non-polarizable electrodes from the scalp [1, 9, 21], and 2) fluctuations in BOLD signal measured by fMRI [4]. In our own studies in the 1970s, we observed similar infra-slow oscillations of extra-cellular oxygen measured by a polarographic method utilizing polarizable electrodes implanted into the brains of neurological and psychiatric patients for diagnosis and therapy [13]. In our studies, we compared these oscillations of extracellular oxygen with local blood flow, impedance and electrical potentials in the infra-low frequency band and determined that these oscillations reflect complex metabolic processes in the brain associated with oxygen consumption by neuronal networks and local blood flow regulation in brain tissue [13].
At the same time, the feedback procedures that utilize infra-slow electrical potentials and HRV as feedback parameters are very well established. The positive effect on symptoms of anxiety, depression, and sleep disturbances has been demonstrated for both techniques [36, 47].
The goal of this paper was to test the specificity of ILF neurofeedback for producing changes in the infra-slow EEG signals of the human brain. It was indeed demonstrated that 20 sessions of ILF training significantly enhanced the amplitude of infra-slow oscillations in brain electric potentials, whereas 20 sessions of HRV training did not change the amplitude of these oscillations significantly. These results support our hypothesis about the direct influence of ILF NF on the objective physiological parameters reflecting brain function.
Little is known regarding the link between the physiological state of the brain and the power/amplitude of infra-slow electrical potentials. According to 50 years of experience of studying infra-slow processes in the human brain under various conditions Valentina Iliukhina from the N.P. Bechtereva Institute of the Human Brain of the Russian Academy of Sciences has suggested that enhancement of the metabolic activity of the brain is accompanied by an increase of EEG power in the infra-low frequency band [57]. Taking this statement into account, we may speculate that 20 sessions of ILF training specifically improve the metabolic activity of the brain.
Nonetheless, the lack of a placebo group, the need for larger randomized samples, and additional assessment measures were the limitations of the present study. Placebo-controlled trials carried out in varied clinical settings with larger groups of subjects could place evidence for the influence of ILF NF on brain electric potentials on sounder footing, although the use of sham treatment raises ethical concerns and even questions of feasibility. More studies comparing ILF NFto an adequate control condition is needed to concern that the ILF NF training is responsible for observed changes of the infra-slow EEG oscillations. However, as the first study of this kind, this research will hopefully stimulate more interest in the scientific community to further investigations of the effects of ILF NF on brain function.
Ethics Statement
This study was carried out in accordance with the recommendations of the ethics committee of the Institute of Human Brain of the Russian Academy of Sciences, Saint Petersburg. All subjects gave written informed consent in accordance with the Declaration of Helsinki.
Conflict of Interest Statement
The authors declare that the research was conducted in the absence of any commercial or financial relationships that could be construed as a potential conflict of interest.
Author Contributions
VAG-Y recruited subjects, participated in experimental design, data acquisition, interpretation, and drafting of the manuscript. OK was involved in the drafting of the manuscript. SAE performed the statistical analysis. MG analyzed data. SO critically revised manuscript and gave final approval. JDK supervised the study and was involved in study design, interpretation of data, and critical review of the manuscript.
Acknowledgments
The authors thank Susan Othmer, Clinical Director of the EEG Institute, Woodland Hills, California, and Dr. Bernhard Wandernoth of BEE Medic, engineer, and head of development of the Cygnet Neurofeedback system used in this study, for their support.
- Aladjalova NA (1956) Infra-slow rhythmic changes of the brain electrical potential. Biophysica 1:127-136.
- Aladjalova NA (1964) Slow electrical processes in the brain. Progr Brain Res 7:156–206.
- Biswal B, Yetkin FZ, Haughton VM, Hyde JS (1995) Functional connectivity in the motor cortex of resting human brain using echo-planar MRI. Magn Reson Med 34:537-541.
- Damoiseaux JS, Rombouts SA, Barkhof F, et al. (2006) Consistent resting-state networks across healthy subjects. Proc Natl AcadSci U S A 103:13848–13853.
- Vanhatalo S, Palva JM, Holmes MD, et al. (2004) Infraslow oscillations modulate excitability and interictal epileptic activity in the human cortex during sleep. Proc Natl AcadSci U S A 101: 5053–5057.
- Bechtereva NP (1974) DC changes associated with the sleepwakefulness cycle. In: Handbook of Electroencephalography and Clinical Neurophysiology. 1974th ed. Remond A: Amsterdam: Elsevier: 10(A):25-32.
- McCallum WC, Cooper R, Pocock PV (1988) Brain slow potential and ERP changes associated with operator load in a visual tracking task. Electroencephalogr Clin Neurophysiol 69: 453- 468.
- Birbaumer N, Elbert T, Canavan A, Rockstroh B (1990) Slow potentials of the cerebral cortex and behavior. Physiol Rev 70: 1-41.
- Bauer H (1998) Slow potential topography. Behav Res Meth Instr 30: 20-33.
- Ilyukhina VA (2004) Human brain in the mechanisms of information and control of interactions of the organism and the environment. Saint Petersburg: Russian Academy of Science, Institute of the Human Brain.
- De Luca M, Beckmann CF, De Stefano N, et al. (2006) fMRI resting-state networks define distinct modes of long-distance interactions in the human brain. Neuroimage 29:1359–1367.
- Bekhtereva NP, Bondarchuk AN, Smirnov VM, Trokhachev AI (1967) Physiology and pathophysiology of the deep structures of the human brain. Leningrad: Medicine.
- Gretchin VB, Kropotov YD (1979) Infraslow non-electric rhythms of the human brain. Leningrad: Nauka.
- Ilyukhina VA, Dambinova SA (2003) Physiological and biochemical principles of infra-slow systems of regulation of states and integrative functions of the central nervous system. In: 2003rd ed. Saint Petersburg State University: Successes of functional neurochemistry: 167-176.
- Vincent JL, Patel GH, Fox MD, et al. (2007) Intrinsic functional architecture in the anesthetized monkey brain. Nature 447: 83-86.
- Nikulin VV, Fedele T, Mehnert J, et al. (2014) Monochromatic ultra-slow (~0.1 Hz) oscillations in the human electroencephalogram and their relation to hemodynamics. Neuroimage 97: 71-80.
- Palva JM, Palva S (2012) Infra-slow fluctuations in electrophysiological recordings, blood-oxygenation-level- dependent signals, and psychophysical time series. Neuroimage 62: 2201- 2211.
- Pfurtscheller G, Schwerdtfeger AR, Seither-Preisler A, et al. (2017) Brain–heart communication: Evidence for “central pacemaker” oscillations with a dominant frequency at 0.1Hz in the cingulum. ClinNeurophysiol 128:183-193.
- Noordmans HJ, van Blooijs D, SieroJCW, et al. (2018) Detailed view on slow sinusoidal, hemodynamic oscillations on the human brain cortex by Fourier transforming oxy/deoxy hyperspectral images. Hum Brain Mapp 39:3558–3573.
- Rockstroh B, Elbert T, Canavan A, et al. (1989) Slow cortical potentials and behavior. 2nd ed. Baltimore: Urban & Schwarzenberg
- Ilyukhina VA, Borodkin YC, Lapina IA (1983) Infra Slow control system of brain and memory. Leningrad: Nauka.
- Beсhtereva NP, Medvedev SV, Krol EM (1984) Investigation of the connectivity of distantly located neural populations of the human brain in the implementation of mental activity. Fiziol J USSR 70: 892-903.
- Mitra A, Kraft A, Wright P, et al. (2018) Spontaneous infraslow brain activity has unique Spatio-temporal dynamics and laminar structure. Neuron 98: 297-305.
- Low MD, Swift SJ (1971) The contingent negative variation and the "resting" D.C. potential of the human brain: effects of situational anxiety. Neuro psychologia 9: 203-208.
- Broyd SJ, Helps SK, Sonuga-Barke EJ (2011) Attention-induced deactivations in very low-frequency EEG oscillations: differential localization according to ADHD symptom status. PLoS One6(3): e17325
- Meda SA, Wang Z, Ivleva EI, et al. (2015) Frequency-Specific Neural Signatures of Spontaneous Low-Frequency Resting-State Fluctuations in Psychosis: Evidence From Bipolar-Schizophrenia Network on Intermediate Phenotypes (B-SNIP) Consortium. Schizophr Bull 41: 1336-1348.
- Guo X, Chen H, Long Z, et al. (2017) Atypical developmental trajectory of local spontaneous brain activity in autism spectrum disorder. Sci Rep 7: 39822.
- Egorova N, Veldsman M, Cumming T, Brodtmann A (2017) Fractional amplitude of low-frequency fluctuations (fALFF) in post-stroke depression. Neuroimage Clin 16: 116-124.
- Kowalski JT, Falkenhagen F, Radtke Y, et al. (2018) Contingent negative variation in acute trauma patients: A prospective exploratory study. Biol Psychol 138: 126-132.
- Reid PD, Daniels B, Rybak M, et al. (2002) Cortical excitability of psychiatric disorders: reduced post-exercise facilitation in depression compared to schizophrenia and controls. Aust N Z J Psychiatry 36: 669-673.
- Badawy RA, Loetscher T, Macdonell RA, Brodtmann A (2012) Cortical excitability and neurology: insights into the pathophysiology. FunctNeurol 27: 131-145.
- Tommasin S, Mascali D, Gili T, et al. (2017) Task-Related Modulations of BOLD Low-Frequency Fluctuations within the Default Mode Network. Front Phys 5: 31.
- Legarda SB, McMahon D, Othmer S, Othmer S (2011) Clinical neurofeedback: case studies, proposed mechanism, and implications for pediatric neurology practice. J Child Neurol 26:1045-1051.
- Van Putten MJAM, Tjepkema-Cloostermans MC, Hofmeijer J (2015) Infraslow EEG activity modulates cortical excitability in postanoxic encephalopathy. J Neurophysiol 113: 3256–3267.
- Othmer S, Othmer SF, Legarda S (2011) Clinical neurofeedback: Training brain behavior. Treatment strategies - Pediatric Neurology and Psychiatry 2: 67-73.
- Othmer S, Othmer SF (2009) Post-traumatic stress disorder—the neurofeedback remedy. Biofeedback 37:24-31.
- Grin-Yatsenko VA, Othmer S, Ponomarev VA, et al. (2018) Infra-Low Frequency Neurofeedback in Depression: Three case studies. NeuroRegulation 5: 30-42.
- Othmer S, Othmer SF, Kaiser DA, Putman J (2013) Endogenous neuromodulation at infra-low frequencies. Semin Pediatr Neurol 20: 246-257.
- Smith ML, Collura TF, Ferrera J, de Vries J (2014) Infra-slow fluctuation training in clinical practice: A technical history. NeuroRegulation 1: 187-207.
- Fox MD, Raichle ME (2007) Spontaneous fluctuations in brain activity observed with functional magnetic resonance imaging. Nature Rev Neurosci 8: 700-711.
- Marshall L, Mölle M, Fehm HL, Born J (2000) Changes in direct current (DC) potentials and infra-slow EEG oscillations at the onset of the luteinizing hormone (LH) pulse. Eur J Neurosci 12: 3935–3943.
- Othmer S, Othmer SF (2016) Infra-low frequency neurofeedback for optimum performance. Biofeedback 44: 81-89.
- Vaschillo EG (1984) Dynamics of slow-wave cardiac rhythm structure as an index of the functional state of an operant. Dissertation, Saint Petersburg, Russia: Institute of Experimental Medicine.
- Lehrer PM, Vaschillo EG, Vaschillo B (2000) Resonant frequency biofeedback training to increase cardiac variability: rationale and manual for training. ApplPsychophyisol Biofeedback 25: 177-191.
- Vaschillo EG, Lehrer PM, Rishe N, Konstantinov M (2002) Heart Rate Variability Biofeedback as a Method for Assessing Baroreflex Function: A Preliminary Study of Resonance in the Cardiovascular System. ApplPsychophysiol Biofeedback 27: 1–27.
- Suh S, Ellis RJ, Sollers JJ et al. (2013) The effect of anxiety on heart rate variability, depression, and sleep in chronic obstructive pulmonary disease. J Psychosom Res 74: 407-413.
- Lehrer PM, Gevirtz R (2014) Heart rate variability biofeedback: how and why does it work? Front Psychol 5: 756.
- Hayano J, Yuda E (2019) Pitfalls of assessment of autonomic function by heart rate variability. J Physiol Anthropol 38: 3.
- HenjeBlom E, Olsson EM, Serlachius E, et al. (2010) Heart rate variability (HRV) in adolescent females with anxiety disorders and major depressive disorder. Acta Paediatr 99: 604-611.
- Jiang XL, Zhang ZG, Ye CP, et al. (2015) Attenuated or absent HRV response to the postural change in subjects with primary insomnia. Physiol Behav 140: 127-131.
- Shinba T (2017) Major depressive disorder and generalized anxiety disorder show different autonomic dysregulations revealed by heart-rate variability analysis in first-onset drug-naïve patients without comorbidity. Psychiatry Clin Neurosci 71: 135- 145.
- Kara O, Polo O (2014) Autonomic and central stress-regulation disintegration in stress-related anxiety disorders. Acta Neuropsychol 12: 1-25.
- Kropotov J (2009) Quantitative EEG, event-related potentials, and Neurotherapy. San Diego, CA: Academic Press/Elsevier.
- Vigario RN (1997) Extraction of ocular artifacts from EEG using independent component analysis. Electroencephalogr Clin Neurophysiol 103: 395-404.
- Jung TP, Makeig S, Westerfeld M, et al. (2000) Removal of eye activity artifacts from visual event-related potentials in normal and clinical subjects. Clin Neurophysiology 111: 1745–1758.
- Othmer SF (2017) The Protocol guide for neurofeedback clinicians. 6th ed. Los Angeles, CA: EEG Info.
- Ilyukhina VA (2013) Ultraslow information control systems in the integration of life activity processes in the brain and body. Hum Physiol 39: 114-126. In Russian.
Figures at a glance